Abstract¶
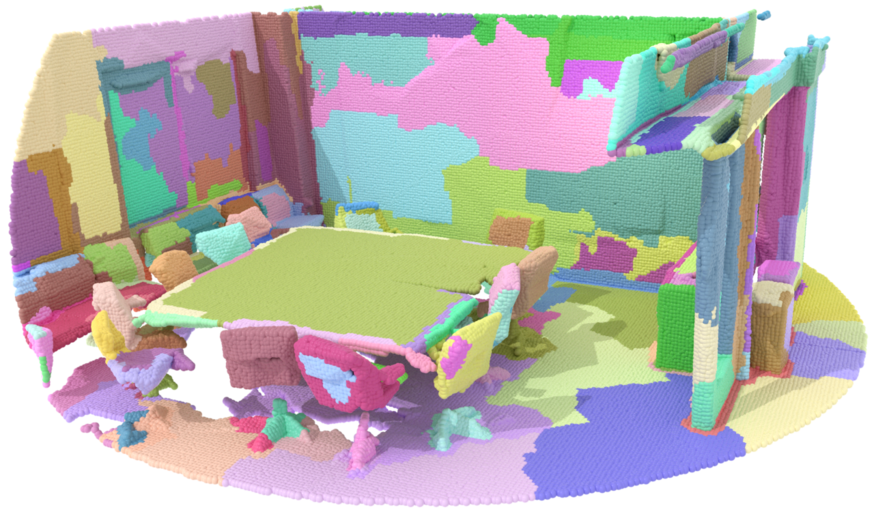
We introduce a novel superpoint-based transformer π€ architecture for efficient β‘ semantic segmentation of large-scale 3D scenes. Our method incorporates a fast algorithm to partition point clouds into a hierarchical superpoint structure π§©, which makes our preprocessing 7 times faster than existing superpoint-based approaches. Additionally, we leverage a self-attention mechanism to capture the relationships between superpoints at multiple scales, leading to state-of-the-art performance on three challenging benchmark datasets: S3DIS (76.0% mIoU 6-fold), KITTI360 (63.5% on Val), and DALES (79.6%). With only 212k parameters π¦, our approach is up to 200 times more compact than other state-of-the-art models while maintaining similar performance. Furthermore, our model can be trained on a single GPU in 3 hours β‘ for a fold of the S3DIS dataset, which is 7Γ to 70Γ fewer GPU-hours than the best-performing methods. Our code and models are accessible at github.com/drprojects/superpoint_transformer